Machine learning ethics and bias - is it a bad thing?
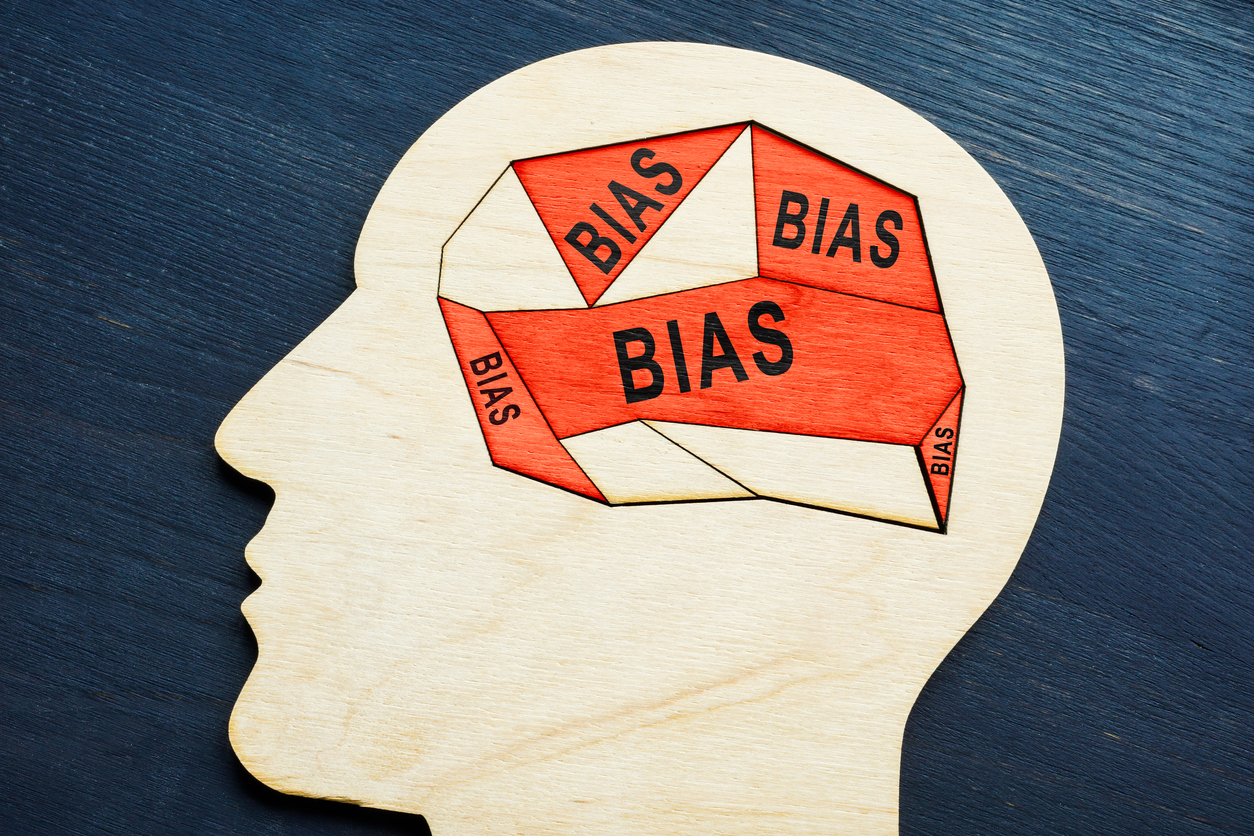
The ethics of machine learning refers specifically to the questions of morality surrounding the outputs of machine learning models that use data, which come with their ethical concerns.
Machine learning has already been used to develop intelligent systems that have been able to predict mortality risk and length of life from health biomarkers. AI has been used to analyze data from EHR to predict the risk of heart failures with a high degree of certainty.
Moreover, machine learning can be used to determine the most effective medication dosage learning on patient real-world and clinical data, reducing healthcare costs for the patients and providers. AI can not only be used in determining dosage but also in determining the best medication for the patient.
As the genetic data becomes available, medications for conditions such as HIV and diabetes will accommodate for variations among races, ethnicities, and individual responses to particular drugs. Within the same data, medication interactions and side effects can be tracked. Where clinical trials and requirements for FDA approval look at a controlled environment, big real-world data provide us with real-time data such as medication interactions and the influence of demographics, medications, genetics, and other factors on outcomes in real time.
As the limitations of technology are tested, there are ethical and legal issues to overcome.
Machine bias
Machine bias refers to the way machine learning models exhibit bias. Machine bias can be the result of several causes, such as the creator’s bias on data used to train the model. Biases are often spotted as first-time problems with subtle and obvious ramifications. All machine learning algorithms rely on a statistical bias to make predictions about unseen data. However, machine bias reflects a prejudice from the developers of the data.
The capabilities of AI regarding speed and capacity of processing far exceed that of humans. Therefore, it cannot always be trusted to be fair and neutral. Google and its parent company, Alphabet, are leaders when it comes to AI, as seen with Google’s Photos service, where AI is used to identify people, objects, and scenes. But it can still go wrong, such as when the search engine showed insensitive results for comparative searches for white and black teenagers. Software used to predict future criminals have been demonstrated to show bias toward black people.[120]
Artificially intelligent systems are created by humans, who are biased and judgmental. If used correctly, and by those who positively want to affect humanity’s progress, AI will catalyze positive change.
Data bias
Bias refers to a deviation from the expected outcome. Biased data can lead to bad decisions. Bias is everywhere, including the data itself. Minimize the impact of biased data by preparing for it. Understand the various types of bias that can creep into your data and effect analysis and decisions. Develop a formal and documented procedure for best practice data governance.
Human bias
For as long as humans are involved in decisions, a bias will always exist. Microsoft’s infamous AI chatbot Tay interacted with other Twitter users and learned from its interactions with others. Once the Twittersphere seized hold of Tay, trolls steered the conversation from positive interactions to interactions with trolls and comedians. Within hours, Tay was tweeting sexist, racist, and suggestive posts. Sadly, Tay only lived for 24 hours. This experiment raises the question of whether AI can ever really be safe if it learns from human behavior.[121]
Intelligence bias
Machine learning models are only as good as the data they are trained on, which often results in some form of bias. Human thinking AI systems have demonstrated bias amplification and caused many data scientists to discuss the ethical use of AI technology. Early thinking systems built on population data showed significant signs of bias regarding sex, race, social standing, and other issues.
An infamous example of algorithmic bias can be found in criminal justice systems. The Correctional Offender Management Profiling for Alternative Sanctions (COMPAS) algorithm was dissected in a court case about its use in Wisconsin. Disproportionate data on crimes committed by African Americans were fed into a crime prediction model, which then subsequently output bias toward people from the black community. There are many examples and definitions of biased algorithms.[122]
Algorithms that assess home insurance risk, for instance, are biased against people who live in particular areas based on claim data. Data normalization is key. If data is not normalized for such sensitivities and systems not properly validated, humanity runs the risk of underrepresenting and skewing machine learning models for minorities and underrepresenting many groups of people.
Removing bias does not mean that the model will not be biased. Even if an absolute unbiased model were to be created, we have no guarantee that the AI won’t learn the same bias that we did.
Bias correction
Bias correction begins with the acknowledgment that bias exists. Researchers began discussions on machine learning ethics in 1985 when James Moor defined implicit and explicit ethical agents.[123] Implicit agents are ethical because of their inherent programming or purpose. Explicit agents are machines given principles or examples to learn from to make ethical decisions in uncertain or unknown circumstances.
Overcoming bias can involve post-processing regarding calibration of our model. Classifiers should be calibrated to have the same performance for all subgroups of sensitive features. Data resampling can help smoothen a skewed sample. But, for many reasons, collecting more data is not very easy and can cause budgetary or time problems.
The data science community must actively work to eliminate bias. Engineering must honestly question preconceptions toward processes, intelligent systems, or how bias may expose itself in data or predictions. This can be a challenging issue to tackle, and many organizations employ external bodies to challenge their practices.
Diversity in the workplace is also preventing bias from creeping into intelligence. If the researchers and developers creating our AI systems are themselves lacking diversity, then the problems that AI systems solve and training data used both become biased based on what these data scientists feed into AI training data. Diversity ensures a spectrum of thinking, ethics, and mind-sets. This promotes machine learning models that are less biased and more diverse.
Although algorithms may be written to best avoid biases, doing so is extraordinarily challenging. For instance, even the motives of the people programming AI systems may not match up with those of physicians and other caregivers, which could invite bias.
Is bias a bad thing?
Bias raises a philosophical question on the premise that that machine learning assumes that biases are generally bad. Imagine a system that is interpreted by its evaluators to be biased, and so the model is retrained with new data. If the model were to output similarly biased results, the evaluation might wish to consider that this is an accurate reflection of the output and hence require a reconsideration of what bias is present.
This is the beginning of a societal and philosophical conflict between two species.