Data science ethics
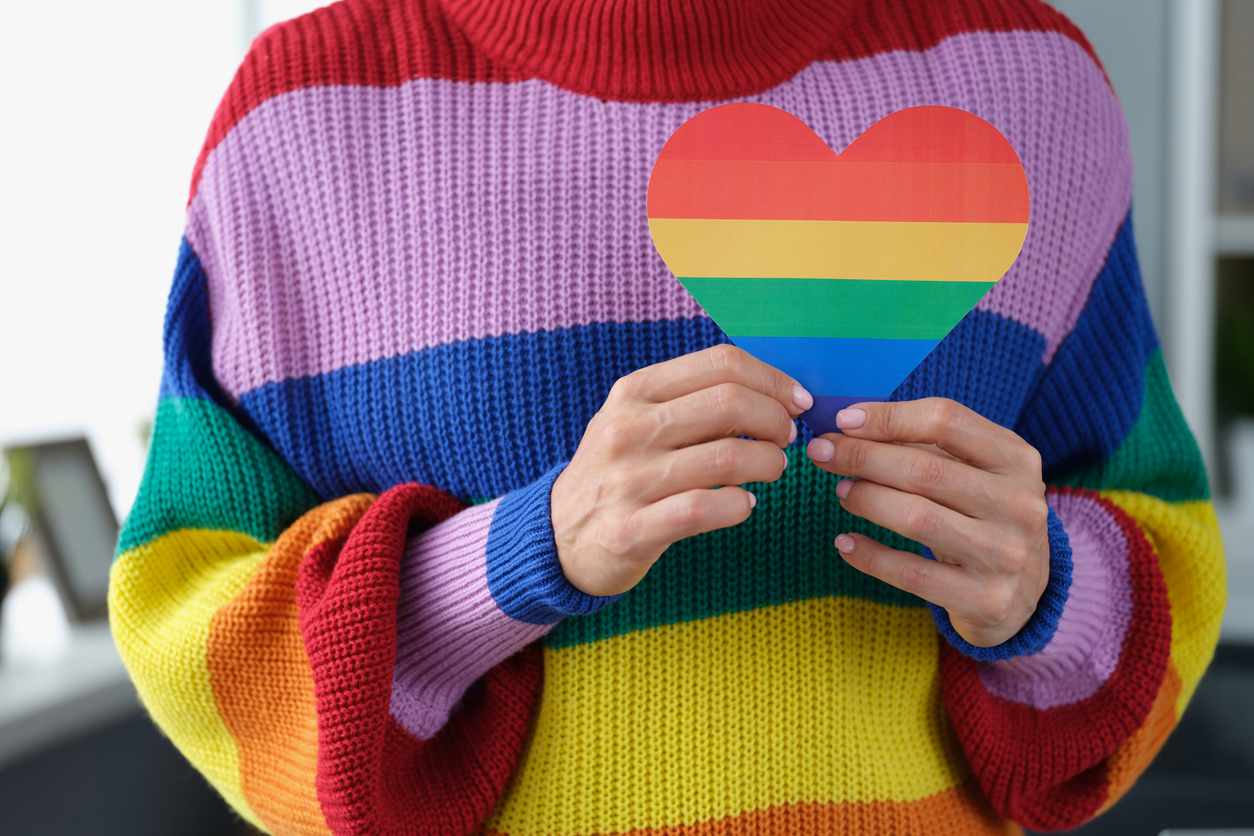
From supermarket checkouts to airport check-ins and digital healthcare to Internet banking, the use of data and AI for decision-making is ubiquitous. There has been an astronomical growth in data availability over the last two decades, fueled by, first, connectivity, and now the Internet of Things.
Traditional data science teams focus on the use of data for the creation, implementation, validation, and evaluation of machine learning models that can be for predictive analytics. Behaviour psychology is embedded within digital health.
From nudges to get app users to submit health data, survey question framing, and presentation of content, to upselling a side of french fries to your fast food app order - machine learning is influencing our decisions, and its influence needs governing.
In the past decade, there has been a wealth of data-driven AI and technological advance specifically in the healthcare domain that have improved quality of life:
- Noninvasive blood glucose levels: There have been many advances in noninvasive blood glucose management. Google has developed a contact lens that determines the user’s blood glucose levels from tears. French company PKvitality developed a small wristwatch that determines the level of glucose in the blood, and there have been advances in determining blood glucose levels through using far-infrared signals.
- Artificial pancreas: As a combination of systems, the artificial pancreas uses two devices. The first device measures blood glucose using a sensor and communicates with a secondary device (or patch), which administers insulin to the patient. The insulin delivery system can adjust the insulin dosage according to the blood glucose levels.
- Reversal/remission of non-communicable disease: digital therapeutics is an emerging discipline of digital health that is redefining conditions such as type 2 diabetes as not having to be chronic and progressive. Digital therapies have been demonstrated to sustain weight loss, improve blood glucose control and place type 2 diabetes and prediabetes into remission.
Novel solutions to problems developed through machine learning are themselves leading to questions of morality and ethics. Currently, governance is moving at the pace of the industry itself. There are many scenarios within AI for which there are no precedents, regulations, or laws.
AI needs to be ethics-based and explainable.
Ethics refers to the moral codes of conduct (or set of moral principles) that shape the decisions people make and their conduct. Morality refers to the principles that distinguish between good/right or bad/wrong behavior.
Ethics in the workplace, for example, is often conveyed through professional codes of conduct for which employees must abide.
Data science ethics
Data science ethics is a branch of ethics that is concerned with privacy, decision-making, and data sharing.
Data science ethics comprises three main strands, which will be covered in further articles:
Ethics of data
This area of data science ethics focuses on the generation, collection, use, ownership, security, and transfer of data.
Ethics of intelligence
This area of data science ethics covers the output or outcomes from predictive analytics that data is used to develop.
Ethics of practices
The ethics of practices was proposed by Floridi and Taddeo, referring to the morality of innovation and systems to guide emerging concerns.[106]
The ethical and moral implications of data use are vast, and best demonstrated through an example.
John, type 2 diabetic, aged 30, has a severe hypoglycemic episode and is rushed to the hospital.
John has been taken to the hospital unconscious for treatment. John, a truck driver, was prescribed insulin to treat his type 2 diabetes by his doctor’s advice, which is most likely the cause of his hypoglycemia. Lots of data is generated in the process—both in the hospital, by healthcare professionals, and also on John’s Apple Watch—his heart rate, heart rate variability, activity details, and blood oxygen saturation to look out for signs of a diabetic coma. John’s blood sample is also taken, and his genome identified. Let’s suppose all this data is used, and it’s useful.
John’s Apple Watch was used to monitor his heart on the way to the emergency room, through which it was suspected that he has an irregular heartbeat, later confirmed with the hospital’s medical equipment.
Upon waking from his hypoglycemic episode, John is pleased to learn that genetic testing does not always give bad news, as his risks of contracting prostate cancer is reduced because he carries low-risk variants of the several genes known in 2020 to contribute to these illnesses. However, John is told of his increased risks of developing Alzheimer’s disease, colon cancer, and stroke from his genetic analysis.